Thank you! Your submission has been received!
Oops! Something went wrong while submitting the form.
Case Studies
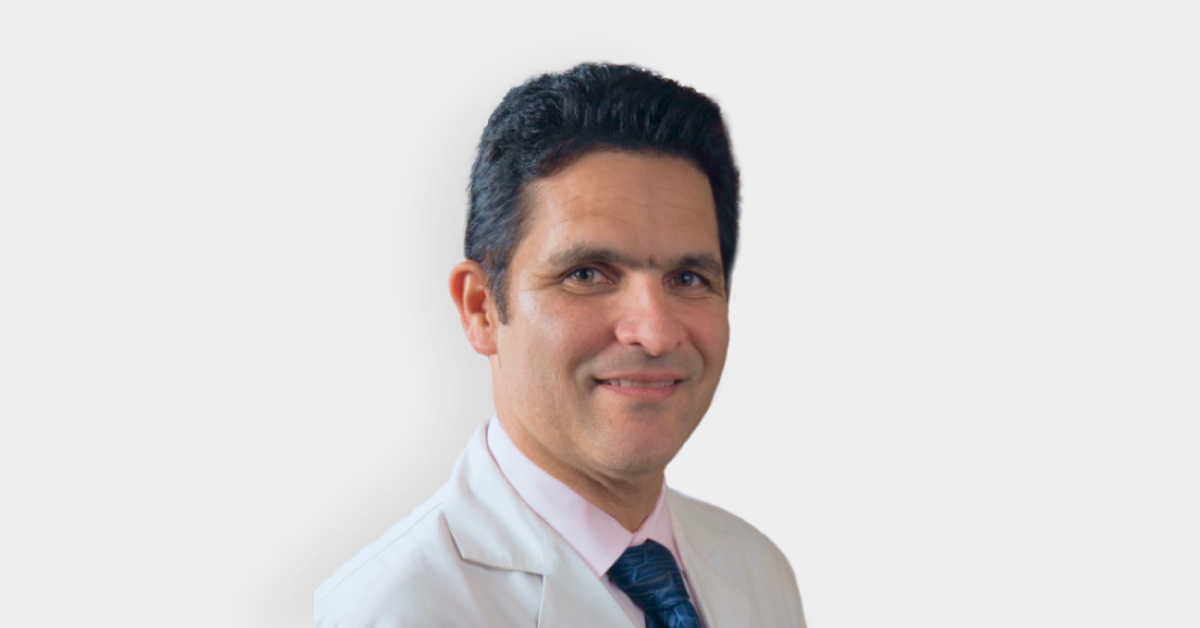
January 29, 2025
Reclaiming Work-Life Balance
Learn how Dr. Lamberty achieved a better work-life balance by using Augmedix Go to enhance patient care and simplify medical documentation.
Media Mentions

January 13, 2025
Augmedix Awarded Vizient Contract for Ambient AI Documentation Solutions
Augmedix, a Commure company, has secured a contract with Vizient, the nation’s largest provider-driven healthcare performance improvement company.
Press Releases
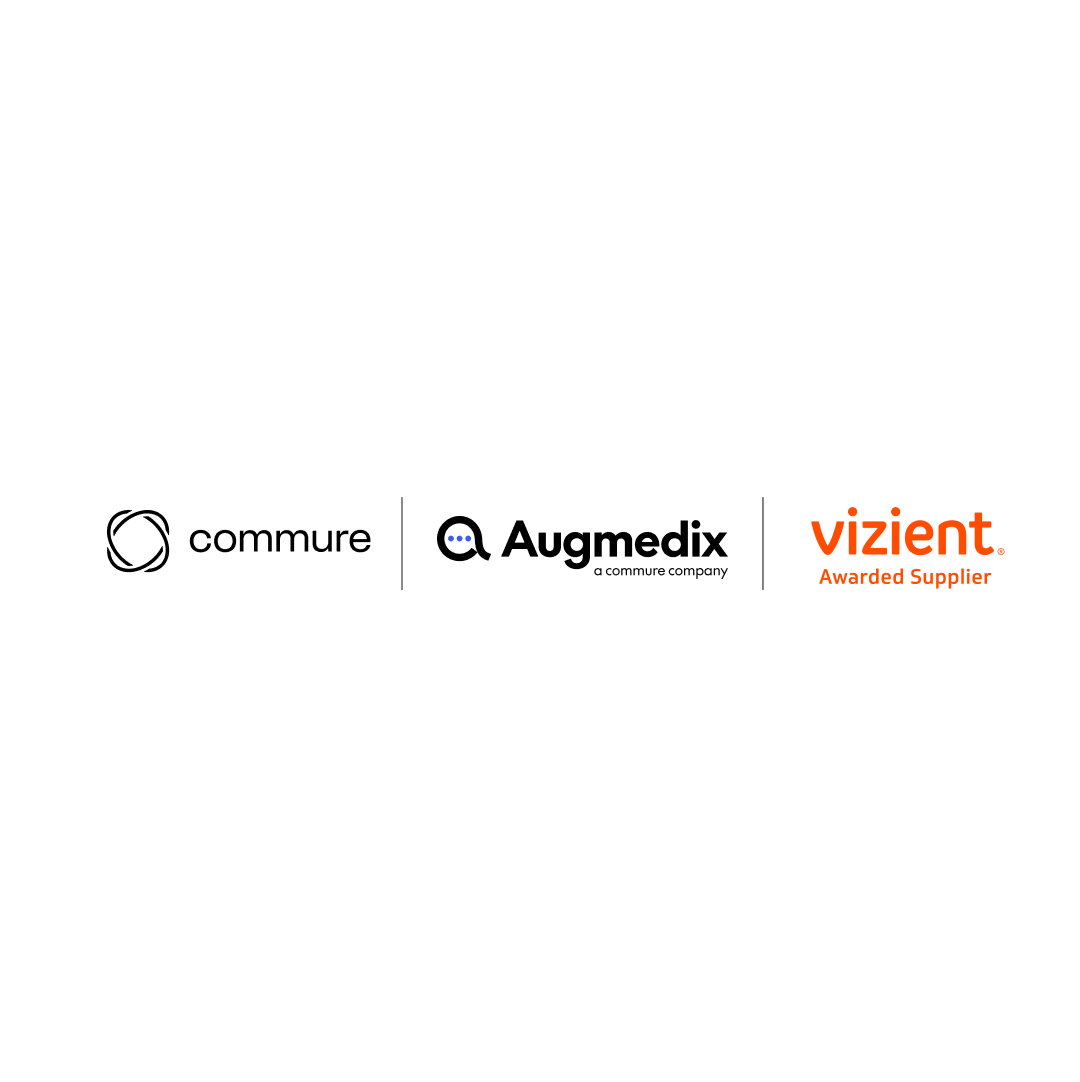
January 13, 2025
Augmedix Awarded Vizient Contract for Ambient AI Documentation Solutions
Augmedix and Vizient to offer Augmedix Go, Augmedix Assist, and Augmedix Live for diverse group of providers and health systems across the United States.
Press Releases
.png)
December 4, 2024
Augmedix Achieves Oracle Validation with Industry Healthcare Expertise for Delivering Proven, Repeatable Integration
Augmedix offers ambient AI medical documentation solutions for both acute and ambulatory clinicians, helping curb burnout, reduce fractional quitting, improve the patient experience
Blogs

November 26, 2024
AMA Coding Overhaul: A New Era for AI Medical Documentation in the ED
AI can have a transformative impact on emergency departments by automatically pulling data from the EHR and patient visits to automatically complete the majority of patient notes.
Case Studies
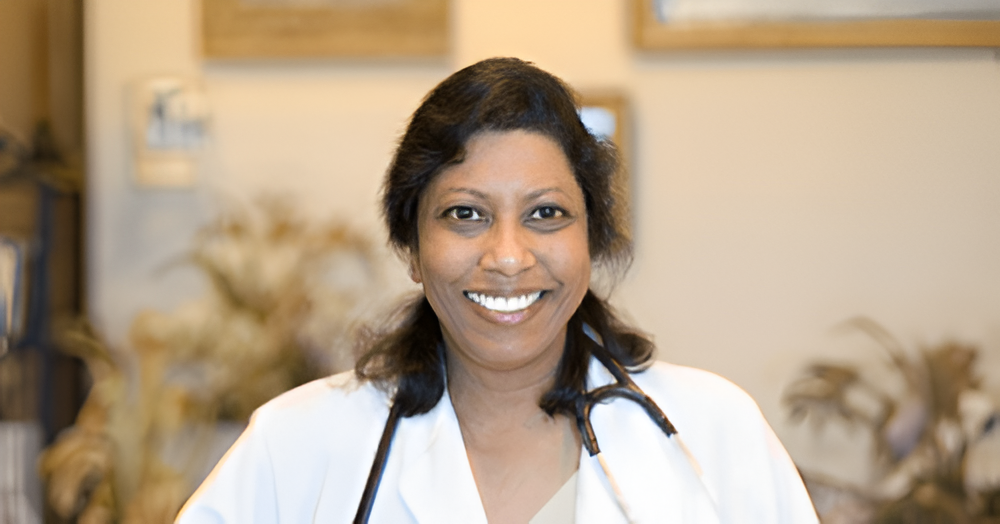
November 12, 2024
Enhancing Efficiency and Patient Engagement
Press Releases
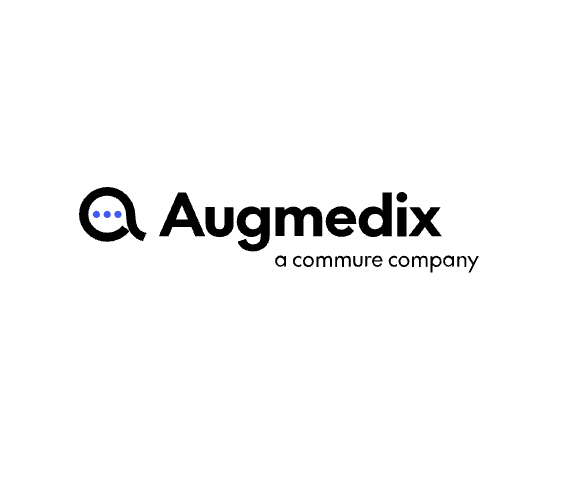
October 2, 2024
Commure Completes Merger with Augmedix
Commure, a healthcare technology company, announced today that it has completed the acquisition of Augmedix. Augmedix will now operate as a wholly-owned subsidiary of Commure.
Media Mentions

August 26, 2024
Ambient AI Is Having Its ‘Moment’ In Healthcare
Forbes highlights Augmedix as an example of using an ambient dictation platform to automatically generate patient encounter notes.
Media Mentions
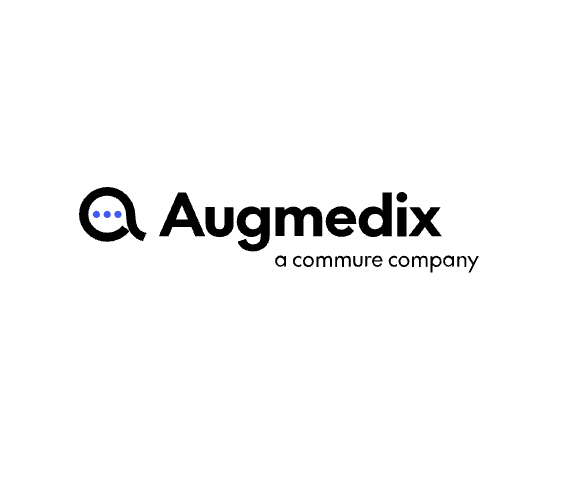
August 24, 2024
Ambient AI Is Having Its ‘Moment’ In Healthcare
Ambient intelligence, or ambient AI, has increasingly become more pervasive across industries and especially become more relevant in healthcare.
Media Mentions
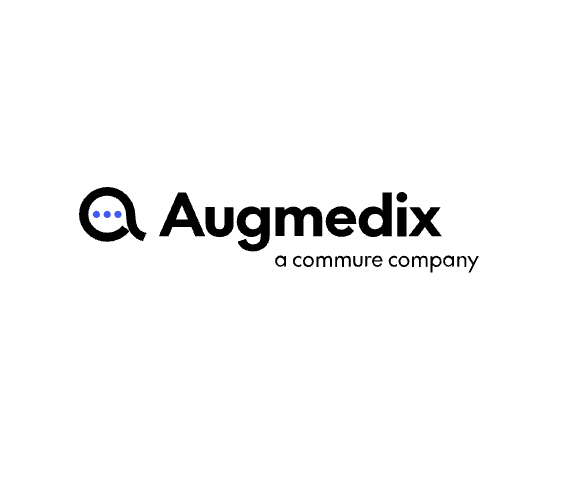
August 21, 2024
Top Artificial Intelligence Companies In Healthcare To Keep An Eye On
Read about the biggest artificial intelligence companies in healthcare ranging from start-ups to tech giants to keep an eye on in the future.
Webinars

August 16, 2024
From Google Glass to AI Scribes: The Augmedix Journey
Ian Shakil discusses the evolution and impact of ambient scribing technology in healthcare, highlighting the company’s journey, innovations, and future directions.
Webinars

August 16, 2024
From Google Glass to AI Scribes: The Augmedix Journey | Ian Shakil | Founder, Director, Chief Strategy Officer of Augmedix
The Evolution of Augmedix: Past, Present, and Future
Media Mentions
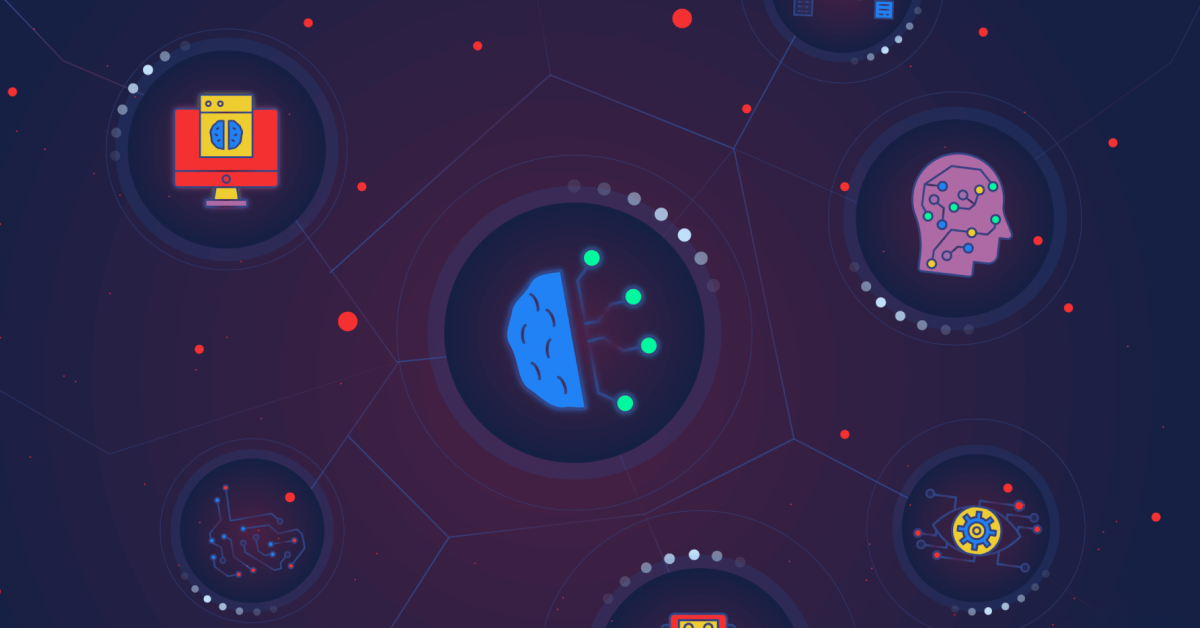
August 13, 2024
Augmedix Named a Top AI Company In Healthcare
Medical Futurist highlighted Augmedix as an industry leader using AI to automate medical administration.
Webinars

August 7, 2024
Healthcare AI Solutions
Augmedix CEO Manny Krakaris discusses the company’s innovative approach to healthcare documentation and recent acquisition by Commure.
Webinars

August 6, 2024
Manny Krakaris, CEO of Augmedix, on the VatorNews podcast
Steven Loeb and Bambi Francisco Roizen interview Manny Krakaris, CEO of Augmedix, recently acquired for $139 million in an all-cash deal, specializing in ambient AI medical documentation